In the application of AI in healthcare, we still have a hard nut to crack. The big question is...
Who is going to pay - and how much - for AI in healthcare?
Technological advancements in healthcare have raised our standard of care, but at the same time raised the costs to deliver that care. A recent Dutch report concluded that the net effect of technological innovation in healthcare is cost increasing, which is also confirmed by a report from the OECD.
These results force us to be critical about the added value of any new technology we bring in, also AI.
Assessing value of AI
So how do we assess the value of AI (or well, any innovation really)? Fryback and Thornbury developed a hierarchical model back in 1991 that describes the different levels on which an innovation (originally focused on medical imaging) may have its effect.
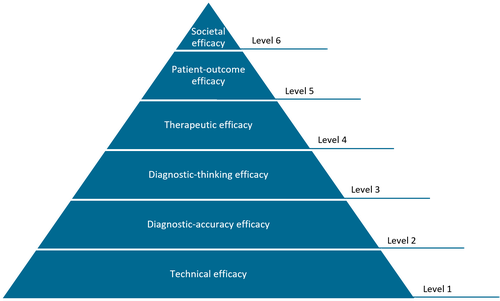
Hierarchical model of efficacy by Fryback and Thornbury, 1991
The levels can also be used to appraise the evidence of AI applications. A level 1 study would, for example, assess whether the product does what it is supposed to do (e.g. works on data from different machines). Level 2 deals with the stand-alone performance, while levels 3-5 focus on the impact of the tool on the follow-up, treatment and outcome of the patient. On top of the pyramid is level 6: the societal efficacy. Does this innovation benefit society as a whole?
In the end, we all want level 6 impact with our technology, making the population healthier while keeping healthcare affordable. So are we there yet with AI in radiology?
Well, we still know very little about that. In a recent study, we looked at the evidence of 100 CE marked AI products in radiology and classified it according to these levels. For only two products studies were performed that evaluated societal efficacy (level 6). Similarly, researchers from Germany concluded that little is known about the clinical impact of AI in healthcare.
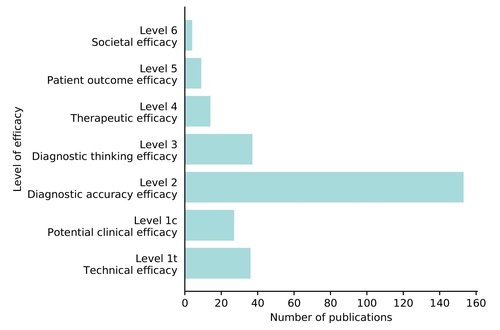
The scientific evidence review resulted in 239 included papers representing 36 out of 100 AI products. A single paper could address multiple levels. Read the full article here.
Estimating the value
The question arises what to do about the lack of evidence of clinical impact of AI. First, I would like to point out that I don't think we should do randomized clinical trials and aim to have level 6 studies for every AI product on the market. That would just as well drive up the costs (maybe a topic to elaborate on in a future post).
There are other approaches to estimate the expected impact and help you answer that initial question: who is going to pay - and how much - for AI in healthcare?
Business case vs HTA
There are two ways to calculate whether an (AI) innovation would be worth it. Either by calculating the business case or performing a health technology assessment (HTA). The Dutch Ministry of Health, Welfare and Sport commissioned the creation of a roadmap to guide the decision and process.
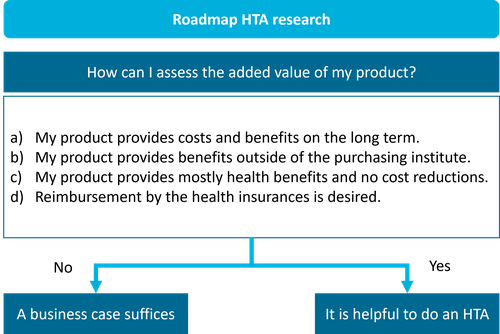
Roadmap to guide health technology research. This is a simplified and translated version of the roadmap provided by The Dutch Ministry of Health, Welfare and Sport which you can find here.
Our hospital was interested in using AI to aid radiologists in the diagnosis of stroke patients. The AI software aids the detection of large vessel occlusions on brain CT scans and should reduce the number of missed occlusions, resulting in on-time treatment and better long-term patient outcomes.
From this very brief summary, you can already see that we are answering the roadmap questions with ‘Yes’ and a business case would not suffice. Therefore, we decided to do an early health technology assessment resulting in our recent publication in Insights into Imaging. †
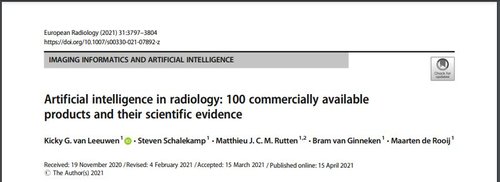
Read the full article published in Insights into Imaging here †.
Early HTA for AI in stroke
By doing an early HTA, one aims to model the potential cost-effectiveness of the innovation before implementing it in clinical practice. This is where it is different from traditional cost-effectiveness studies where you look back after implementation to calculate the impact on costs and/or health.
So before throwing our money at buying, reimbursing, or even starting the development of an AI product, we can already make an assessment of its potential clinical and monetary value.
Dealing with assumptions
The consequence of doing an early HTA is that you have to deal with assumptions. You do not know yet exactly what the outcomes will be. Though, the advantage is that you can play around with these parameters. What if the price would be lower? Or if the performance of the AI tool would be better? Or if our target population would be different?
For this study, we looked at cost-effectiveness from a societal perspective (one could also do so from e.g. a hospital or regional perspective). We based our population characteristics and treatment costs on previous research from the UK. We assume that 6% of the large vessel occlusions are missed in clinical care and that half of that could be reduced by the AI tool. We set the initial price of the AI at 40$ per patient that would come in with an indication stroke in the emergency department.
In this figure, we vary the price of the AI tool and the performance (the ability to reduce missed occlusions), showing where costs get reduced (in green) and when costs increase (in red).
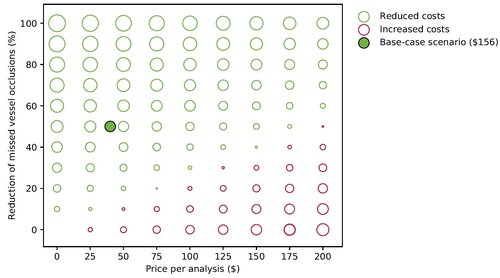
Incremental costs at varying prices for the AI tool per analysis ($0 - $200) and varying percentage of reduction of missed large vessel occlusion diagnosis (0% - 100%). Green circles demonstrate a cost reduction whereas red circles signify an increase in costs. The size of the circle is related to the height of the incremental costs. In the base case scenario the AI tool may cause an average cost reduction of 156$ per patient.
Benefit vs cost allocation
As you can see, in many situations, the costs of healthcare as a whole would be reduced. Where I think it gets interesting though, is when we zoom in on where these benefits and costs are allocated. Because that will bring us closer to that initial question: who is going to pay - and how much - for AI in healthcare?
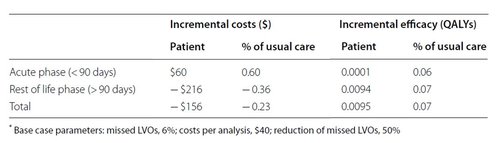
Results of base case analysis as the difference between usual care and applying the AI tool. View the publication for more elaborate version.
In this table, we differentiate the costs and efficacy (health effects) for the acute phase (the first 90 days after the stroke), and the rest-of-life phase. In the short term, per patient, the AI tool will cost 60$ per patient more instead of reducing costs. Also, the health effects are minimal. Only later in life do we see the cost reduction, because a patient may need less returning visits to the hospital, or may have avoided the need for a nursing home.
Taking these results into account, for the radiology department it is not self-evident to buy such an AI tool as it will cost the department more than it gets back. However, with sufficient performance of the AI tool and reasonable pricing it might, from a societal perspective, be worthwhile to roll out.
You can try it out yourself
As said earlier, the nice thing about early HTA is that you can play around with the model variables and easily adapt it to your own situation. To do so, we have made the Excel model publicly available.‡ The main parameters such as population size, price of the AI tool, and amount of missed occlusions can be easily altered on the first page.
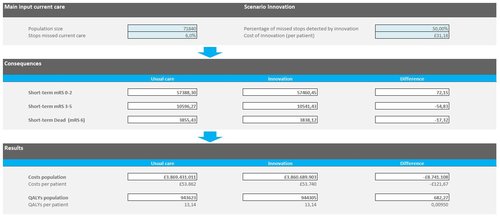
Early HTA model for AI aided occlusion detection made in Excel. You can download the model here to adjust it to your own setting.‡
So what now
Well, I am not arguing that everybody should buy AI for stroke to avoid missed occlusions. It, first of all, really depends on the local clinical context, such as the available (neuro)radiological expertise and the frequency occlusions get missed. Secondly, the software’s capability to prevent missed occlusions (level 3) determines whether it is worth the effort and so far there is no clear evidence of that.
But assessments like these can contribute to answering the question with which we started: who is going to pay - and how much - for AI in healthcare? Is there a solid business case and can departments or hospitals make the investment themselves, or do the benefits lie elsewhere and would reimbursement be more sensible?
In the United States, the health insurance Centers for Medicare & Medicaid Services have taken the first steps in acknowledging this discrepancy of costs and benefits and have introduced some form of reimbursement for specific AI tools §.
Will European countries follow? Probably… Maybe already have? As every country has their own healthcare system, it is difficult to get the overview. Feel free to let me know the status of reimbursements for AI (in radiology) in your country!
† Leeuwen, K. G. Van, Meijer, F. J. A., Schalekamp, S., et al. (2021). Cost‑effectiveness of artificial intelligence aided vessel occlusion detection in acute stroke: an early health technology assessment. Insights into Imaging. https://doi.org/10.1186/s13244-021-01077-4
‡ Download the early HTA model for large vessel occlusion detection in stroke here.
§ Read more in the excellent blog post by Luke Oaden on this complex structure.¶
Main image is a photo by Morgan Housel from Unsplash.